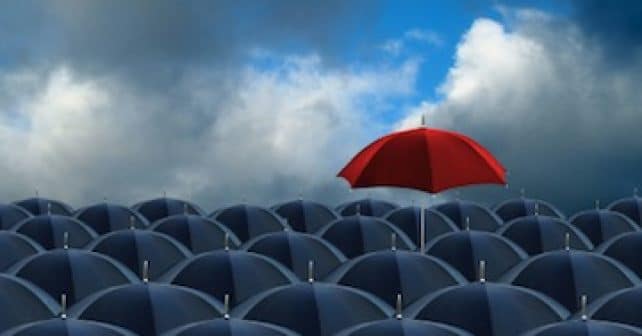
Just over a decade ago, the financial industry set out to reduce credit counterparty and systemic risks. Now with geopolitical change, the Coronavirus and news of constant cyber attacks, risk managers are shifting their focus and budgets to mitigating emerging risks around the rapid adoption of technologies, including DLT, AI, ML and Cloud technologies. While these newer technologies represent great opportunities, they also introduce new risks. In a DerivSource commentary, Kate Scott, partner at Clifford Chance in London shed light on the legal, ethical, and reputational risks that firms should be aware of when adopting these newer technologies, and what they can do to avoid them. Listen to podcast this article is based on here.
Banking litigators traditionally focused on disputes arising out of financial documentation, such as ISDA disputes or mis-selling claims. They still do a lot of that, but litigation and enforcement risk for financial institutions can increasingly arise from the technology they are using – in their internal processes, in targeting their services to clients, or in the products that their customers are buying from them.
These risks might not be immediately obvious. With artificial intelligence (AI) in particular, risks may be embedded in the underlying data or computer code, which legal, compliance and internal audit functions might not necessarily have immediate access to. Financial sector clients need to consider those risks at the development stage, implementing systems, controls and good governance, as well as at the backend, when issues arise. Enforcement actions are driving increased interest in this area, and some firms are considering IT risk at the design and rollout stage. However, most firms are not yet doing enough in this space.
Presently in a financial services context, AI is less about fully autonomous artificial intelligence or machine learning (although there are institutions developing innovative use cases) and more about automation – where data inputs are overlaid with computer code to achieve a particular output. For example, using customer data for credit assessments, using data lakes to identify patterns to make investment decisions and to trade, or using data to target products towards customers and then using algorithms to formulate a trading or investment strategy. With innovation at the top of the financial services agenda, financial sector clients, perhaps more than any other sector, are thinking carefully about future market differentiators.
Legal risks and enforcement
Data issues top the list for AI legal risks. That might be personal data (regulated by the General Data Protection Regulation (GDPR) in the UK and European Union, and the California Consumer Privacy Act in the US), client data (such as information about a client’s trading patterns) or big data.
Global financial regulators have shown their willingness to apply existing regulatory principles to AI. Firms need to be mindful of over-reliance on automation, and they need to think about AI systems’ fitness for purpose, as well as accurate marketing, testing and accuracy. The UK’s Financial Conduct Authority (FCA) can require firms to produce a description of their algorithmic trading strategies within just 14 days. They say that firms should have a detailed algorithm inventory, they should be able to set up coding protocols, usages, responsibilities and risk controls. If a firm does not have this in place already, 14 days is too short to pull that information together. Data regulators, in particular, see financial services as a testing ground, so firms should expect more regulation and enforcement in this space.
Data regulators, in particular, see financial services as a testing ground for AI, so firms should expect more regulation and enforcement in this space.
Market abuse, including using AI to further financial crime, insider trading or market manipulation, will be a major area for enforcement. Similarly, anti-competitive conduct, where firms implement algorithms that drive common customer outcomes will be a focus. The European Commission currently has live investigations looking at data and big tech. Anti-trust data, financial sector and other industry-specific regulators will all bring enforcement actions to demonstrate that they are tough on AI.
AI usage can also have unintended consequences and firms could see claims for breach of contract or in tort as a result. The boundaries of existing terms and conditions and exclusion clauses will be tested. Firms need to make sure their terms remain fit for purpose, particularly where AI is concerned. More claims will be heard in the civil courts, which will have to decide who is liable when an AI powered system causes substantial losses.
Ethics and AI
Tackling bias and discrimination is a very hot topic in 2020. There is always an inherent risk of AI incorporating biased data sets and creating biased outcomes, which can lead to unfair or discriminatory decision making. This is already covered under existing anti-discrimination laws and to some extent, financial regulatory principles, but it can be tricky to spot and enforcement actions are on the rise, bringing reputational risk for firms.
While outright discriminatory practices are illegal, there are no strict laws on the ethical use of AI. Ethical values differ from country to country – they are hugely influenced by cultural considerations and they are continually evolving. Over the last year, hundreds of new ethical frameworks have been published in various jurisdictions. The core themes are fairness, accountability, transparency and human oversight. But the real issue is how firms implement those principles within their businesses. Firms must be able to explain how the AI works to their employees, customers and regulators, in an accessible and transparent way, whether their AI is bought or built in-house.
In a Clifford Chance survey undertaken by The Economist, 88% of board level respondents said they were confident in their ability to manage AI risk. However, when asked what steps they had taken to address AI risk in their business, some 46% had taken no action. That’s a big gap. Firms cannot be confident unless they have looked at these issues and implemented systems and controls.
Practicalities: Governance, diligence and audit
In order to make sure they are using and managing AI in a sound, compliant and ethical way, firms need to think about governance, due diligence and audit.
Governance begins with clear oversight from the board and requires embedding a culture of lawful and ethical AI use, coupled with management responsibility. AI governance frameworks can be stand-alone, they can be AI/ethics, or they can be fitted within existing product approval or other policies, or governance processes. The hardest thing is drawing it all together. Firms need a broad team that includes legal, compliance, as well as business teams, IT specialists and data experts, all working to the same objectives, and they need to do this globally.
A major challenge is that existing policies tend to be quite disparate. There are separate policies for GDPR compliance, human rights, existing competition policies, as well as a code of conduct and a new product approval process. To what extent do those policies already contemplate AI use? And if they do, are they consistent? To what extent could or should they be replaced by an AI governance framework? There is no one-size-fits-all solution.
Due diligence requires getting a handle on existing and proposed AI usage within a business line. Too often, AI usage is siloed. Further, many lawyers and compliance teams don’t know or feel confident about the questions they should be asking those building the technology to flush out the answers and to put in place the processes they need. Upskilling in this area should be a key area of focus.
A key piece of the control structure is undertaking a legal impact assessment to see where the risks are. Firms need to look out for ensuring data set ‘cleanliness’, transparency over how data is being used, sourcing written explanations of the AI’s functionality, monitoring and testing the AI’s decision making as well as ascertaining limits and liabilities. AI is constantly developing, and machine learning programs may even be developing their own functionality. Firms need to audit their systems to make sure their AI is working how it is supposed to, so they are not caught on the back foot when the regulators come.