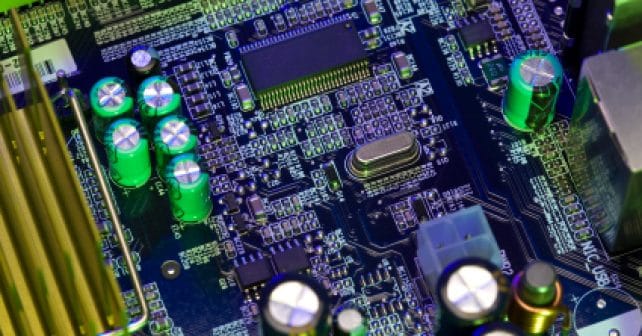
Artificial intelligence, machine learning, robotics process automation—all buzzwords of the moment—are different from one another and how they add value in the world of derivatives. Axel Pierron, co-founder and managing director of capital markets management consultancy Opimas, discusses the current and potential future applications of AI technologies in the derivatives space. (Listen to the podcast interview).
Machine learning and artificial intelligence (AI) are often used as synonyms, but the reality is that they are a bit different. Machine learning is a process in which we teach a machine how to improve itself. When this is done with a supervised role—with a human telling the machine what is right and what is wrong in terms of output—it is not really machine learning but, rather, a rules-based engine. AI has that idea of intelligence, which means the system is trying to mimic how the human brain processes things. In this case, you can have machine learning and, especially, what we call unsupervised machine learning, which is part of AI.
A good example is AlphaGO, a computer program that plays the game Go, developed by Alphabet’s Google DeepMind team in London. When AlphaGo learned how to play Go, it learned to play against different iterations of the AlphaGo program—so it played against itself—and it came up with new strategies that had not been envisioned by human beings. That’s one of the clear elements with AI, that it will allow humans to envision patterns or options that they may not be considering themselves.
Also under the large umbrella of AI and machine learning, we hear a lot about robotic process automation (RPA). However, RPA is really outside of AI. It’s a rules-based engine that is able to replace repetitive manual interventions, and that’s why we often see it being deployed in back-office operations. However, when you combine RPA with AI solutions—such as image recognition, for example—you end up with something called intelligent automation, which clearly is in the AI realm.
AI represents the beginning of a new market infrastructure
AI will have an impact on the whole value chain of capital-markets operations. The question is when will it hit the market? One of the challenges is the complexity of implementing new technology in operations.
Currently, there is a pretty even level of spending in machine learning technology, RPA, and cognitive analytics solutions. RPA has gained a lot of momentum because—especially in the post-trade arena—it’s a low-hanging fruit. RPA is already being implemented and has driven a lot of investment from market participants looking to automate manual back-office processes.
However, we expect those investments to decrease in the mid-term, as the benefits of RPA will be delivered. However, machine learning, cognitive analytics and the overall AI piece will continue to increase in the future as market participants uncover new applications and business opportunities. We are really at the beginning of a new market infrastructure, and market participants are uncovering new applications almost every day.
“An important foundation of AI solutions and machine learning techniques is having big data solutions, and data that is of good quality and well categorized. The cornerstone of any AI strategy therefore is to have a relevant data strategy first, and a big data strategy.”
An important foundation of AI solutions and machine-learning techniques is having big data solutions, and data that is of good quality and well categorized. The cornerstone of any AI strategy therefore is to have a relevant data strategy first, and a big data strategy. Most market participants are still struggling with their big data strategy, and their ability not only to store the data, but also to categorise, cleanse it, etc. That’s what the majority of people are still working on.
The next level of adoption will be around machine-learning technology, where the system acts as a recommendation tool, but cannot conduct or identify trading strategies itself. The most sophisticated market participants are starting to do this, but it’s only a handful of market players. There is a lot of interest because people are identifying the opportunity that AI can represent to the industry, but we are really at the early stage of launching it in practice.
Changing the face of trading
AI will have a significant impact on the trading side, with the ability to leverage new data sources and identify new trading partners, trading options or trading strategies. The most sophisticated market participants are already using this.
AI solutions have the ability to crunch numerous data sources, which are often asymmetric. Firms receive market data on a real-time basis, but there are other sources of data that they use in a trading strategy that may come on a daily, weekly or a bi-monthly basis. For human beings, it is a complex process to recrunch all the different data sources, especially when the timing is irregular. AI offers the opportunity to uncover new trading strategies and trading options. AlphaGO has demonstrated that AI can identify strategies that have not been considered by humans before and that are successful. That is opening up a new world of opportunity for market participants.
The question is when this will become more common. We are seeing some initial implementation of AI in the trading arena, but widespread implementation will take some time, because it is a new technology and the learning process is ongoing. The risk with an AI trading solution is that it could run very well for two to three years, and then, three years from now, you might uncover that there have been some issues, and some trading that was conducted that did not match your expectations. So even as AI is being implemented, firms will need to supervise the activity of their AI.
Potential applications of AI in the derivatives space
There are numerous ways that AI can be used in the derivatives space, especially when you couple that with, on the trading side, new alternative data sources, whether from static imagery, telecommunications, or the shipping industry, for example. AI could certainly provide great value for the most exotic or complex derivatives, whether it’s in terms of pricing, valuations, or extracting information. Mizuho Bank recently launched a project using AI to extract ISDA contract information through image-recognition solutions and to feed that into its system.
One of the big draws of AI is its potential to help improve operational efficiency. This is a very sensitive topic within the industry because it should enable employees to do more with less. Eventually, it will have a dramatic effect on the number of employees per activity, so it will have tremendous impact in terms of employee reduction in back and middle offices and on the trading desks as well.
It will also have a major impact on the overall evaluation of risk and risk management practices, and there it will certainly drive improvements. Also, one of the very interesting implementations of AI is its use to evaluate the efficiency of current processes and identify not only the most common inefficiencies and solve them, but also to find opportunities to improve overall efficiency.
Finally, there will be a tremendous impact on the compliance side. US-based start-up Droit Financial Technologies, for example, leverages AI for derivatives trading and performs advanced risk checks to ensure transactions are compliant, and also does some reporting activity.
Since the financial crisis, the industry has been hammered with numerous new regulations, including EMIR, Dodd-Frank, and MiFID II, and the majority of market participants have responded to this regulatory tsunami by hiring more and more compliance officers and beefing up their legal and compliance teams. While it has allowed the companies to operate under a decent regulatory framework, it has had a major impact on their cost-income ratios. AI in compliance and regulation will therefore have a tremendous impact on the overall cost reduction of financial institutions.