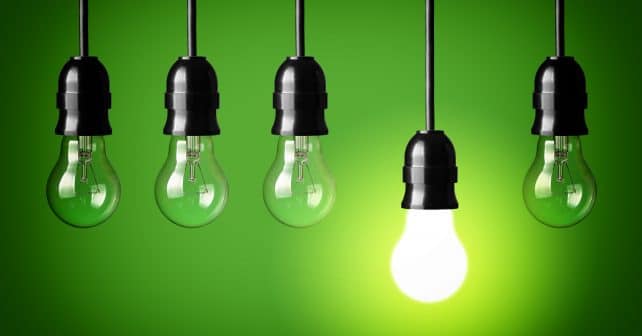
Legacy systems are inadequate for the sorts of complex computations needed to ensure compliance with post financial crisis regulations. In a Q&A, Alexandre Bon, Senior Solution Architect at Murex explains why a regulatory calculation engine is essential for compliance with BCBS’s new market and credit counterparty risk requirements.
Q. What is a regulatory calculation engine?
A. It is basically a centralized risk system that leverages enterprise data management capacities, a unified valuation engine across asset classes and high performance computation facilities to support the capital assessment needs of all departments across the firm.
Between the stream of recent “Basel IV” regulations, the push to central clearing and the upcoming margining rules for uncleared derivatives, there is now a strong focus on improving the management of regulatory capital for the Trading Book. As firms face new regulatory constraints, a skyrocketing cost of capital for their traditional trading activities and increasing demand for collateral assets, they are transforming their business models and placing regulatory capital calculations at the center of their profitability analysis.
This, combined with a massive increase in the complexity of the regulatory computations, means that legacy infrastructures cannot cope with the new supervisory and business requirements. The days are gone when producing the capital reports was a monthly data-crunching exercise run by the finance department. Now risk, the front office, collateral trading and XVA desks all ask for intra-day view of the capital requirements.
The old way of managing capital calculations in legacy regulatory reporting engines is not going to cut it anymore for two reasons. One is the complexity of the calculation and data requirements for market risk, credit risk, and credit valuation adjustment (CVA) charge. That calculation has grown tremendously, and the regulators are not showing signs of slowing down. Second, since capital is becoming such a constraint on the business, institutions need to move towards a more proactive way of managing it. That means much closer views of the regulatory capital position, including real-time sensitivity analysis.
“Firms therefore need a specialized engine—well integrated with the risk management, front office and collateral management applications—that will be responsible for performing the core computations for SA-CCR, FRTB, and initial margin types of calculations.”
Firms therefore need a specialized engine—well integrated with the risk management, front office and collateral management applications—that will be responsible for performing the core computations for SA-CCR, FRTB, and initial margin types of measures.
There are four steps to producing a regulatory capital report. The first is gathering the data, which used to be a monthly or quarterly exercise. Now we need this process to be much closer to real-time.
The second is running the regulatory computations, which have become much more challenging, especially since Basel Internal Models (FRTB, CVA, Credit) and initial margin computations require many VAR-types simulation runs, as well as much more granular data around positions and market data.
The third step is the results aggregation, which is also becoming much more complex under the new regulations. Take the market risk charge under FRTB-IMA for instance: you will now need to compare and aggregate multiple stressed Expected Shortfalls runs (by desk, by risk factor, adjusted for different liquidity horizons), the Default Risk Charge (DRC), as well as the Revised Standardised Approach (RSA) results which will serve as a capital floor. The regulatory calculation engine should perform this aggregation close to real time, and process changes, data corrections and reruns efficiently.
The fourth and last step consists in collecting final results to produce the regulatory reports following the formats and requirements of each supervisor the institutions will report their capital results to. This requires a rich reporting engine downstream.
To fulfill these new business requirements, the first three steps (data collection, risk computations, results aggregation) need to be very closely coupled. This is how a regulation calculation engine can deliver results to each business units much faster while reducing operating costs (eliminating unnecessary intermediary steps and interfaces with the associated reconciliation processes). This way of orchestrating regulatory computations offers significant operational improvements to the capital reporting process, but as well for stress-testing and sensitivity analysis exercises.
Finally, it makes sense to build an infrastructure, which is consistent from a data representation and interpretation view point, and allows you to navigate all the calculations in a consistent manner across these different regulations.
Q. What department would own this calculation engine? Where would it sit within a firm?
A. Which department owns this calculation engine may vary from firm to firm. It could be risk, or even a business unit close to the front office, such as a XVA desk. It could be a shared initiative too. If you make the investment of putting this regulatory calculation engine in place, there is so much benefit for the business, that finance is unlikely to have sole ownership of the engine, especially since other departments will require the same calculations but on an intraday basis.
Q. How can this regulatory calculation engine support a firm’s compliance needs for both FRTB and SA-CCR?
A. To start with, it delivers full transparency over risk and capital calculations, and greatly improves modeling accuracy through consistent valuations and the quality of underlying data.
You need, especially with FRTB, to be able to reconcile the risk and front office P&L calculations. Under the Internal Model Approach, you have to meet back testing and stringent P&L attribution tests. Under the Revised Standardised Approach, you will also need to produce consistent sensitivities across all asset classes and desks (including smile dynamics and index decomposition) for both vanillas and exotics. It becomes critical to be able to reproduce and explain any difference between figures that you have on the regulatory reports and on the front office side. A centralized valuation engine that makes that link is going to be an essential component to achieve compliance and especially retain an internal model approval. Such an engine will let you break down the effects of different market data assumptions (between front office desk and finance, for instance), model settings (pricing routines or curves and surfaces calibration) and analyze variations down to the individual transactions and risk factors.
In the case of SA-CCR, the need differs a bit as the intent of the regulator is specifically to eliminate all dependencies on the Bank’s internal models (except for the Mark to Market input to the Replacement Cost). There remains nonetheless a lot of ambiguity in the Basel rules regarding the mapping of transactions onto the regulatory inputs – especially for complex products. This yields complex data mapping questions as a result: which value should be used as an effective notional of a product with a stop-loss clause? How can I properly recognize a basis transactions when the underlying is a basis index? What is the price and strike I should use to compute the regulatory delta for complex options? To implement accurate and capital-efficient mapping rules for all products consistently, you need a rich trade repository with well-defined transactions and instrument classifications, which can capture all these variations in trade terms, as well as a risk engine that can make sense of them. Legacy data warehouses that were implemented at the time of Basel II are very far from that.
Eventually, you want a framework that delivers a consistent view of positions, P&L, risk metrics, sensitivities and their underlying trades, instruments and market data, across all data sources and asset classes. For all of these, you need a “single version of the truth” be it for finance, risk, and regulatory reporting functions, but as well for XVA and front office desks or the collateral management group. Enterprise-wide capital planning and stress-testing exercises also benefit greatly from the implementation of a central regulatory calculation engine. For stress testing, the same scenarios need to be executed to observe impacts on the credit and market risk charges as well as on the liquidity ratios. Having just one layer for data input and consistent management of the calculations can help tremendously with this exercise. And, of course, regulators will look to see that assumptions and execution are consistent across these different areas.
Lastly, a key objective for the Banks working on their FRTB, SA-CCR or Standard Initial Margin Model (SIMM) calculation and data management infrastructure, is to put in place an adaptable system framework that can let them easily adopt new regulatory requirements as they come in (and constant regulatory updates seem to have become be the new normal) – and do so without massive infrastructure investments. They are also looking for a framework that can handle varying interpretations across regulators and improve the time-to-market of new products and activities. While regulatory checklists and siloed systems have been an obstacle to reactivity in the past, there is now an understanding that risk technology will be a key business enabler. Institutions which can deliver to all stakeholders a real-time consistent view of their capital, collateral and risk positions across the trading book will be the best placed to develop innovative business models.
Q. What about the operational aspects of the regulatory reporting process: does a regulatory calculation engine provide benefits there as well?
Yes, such an engine would certainly improve the efficiency of the whole calculation and reporting process while also delivering a much more complete and robust environment from an audit point of view. Once again, I am thinking of the transparency and data consistency benefits, but as well of how a central engine can tremendously reduce the complexity around data feeds* and simplify all operational processes around data adjustments and re-runs, stress-testing, validation, results analysis and reporting in general.
For instance, we can see that, by design, a central regulatory calculation engine will follow the Completeness¸ Timeliness and Adaptability principles at the heart of the BCBS 239 regulation (“principles for effective risk data aggregation and risk reporting”) that applies to systemically important banks. Also, with reporting requirements coming closer to real time, it is essential to automate manual processes and cut down on lengthy data massaging and reconciliation exercises.
“New internal model calculations — especially for the FRTB and CVA capital charges — are very computationally intensive (…). Firms therefore need to be smart from a hardware management perspective, making sure the calculation is efficient, well distributed, and can be optimized by computing only what is strictly necessary.”
Most importantly, the main benefit the institutions will reap is a significant improvement of computation performances, since the key objective of such an engine is to optimize the calculation chains. The new internal model calculations — especially for the FRTB and CVA capital charges — can be extremely computationally intensive, and some capital-linked profitability metrics like KVA (the lifetime cost of capital) even more so. Institutions desperately need to find smart solutions to distribute and orchestrate calculation and aggregation tasks onto their hardware in the most efficient ways. And this goes beyond simple parallelization of valuation routines over a grid, you also need to load data optimally, avoid redundant tasks, ensure that you compute only what is strictly necessary (when performing sensitivities calculations or looking at liquidity adjusted expected shortfalls, for instance), and feed the aggregation engines more efficiently.
Without this kind of infrastructure, maintaining an internal models approval can easily cost millions in additional hardware, and I don’t even mention the cost of delivering near real-time capital, XVA or Initial Margin computation capacities.
On the cost-saving front, such an engine can help materialize multiple synergies. There are overlaps, for instance, between the standard and the internal model approaches. If your bank has internal model approval, you will still need to compute the SA-CCR because you need to produce it for a number of other regulatory calculations like the large exposure reporting. Also, for both credit and market risk, you will probably need to compute the standardized method as a floor to the internal model part. It is also likely many institutions will only get partial regulatory approval across their portfolio for internal models. For portfolios, which are not approved for the internal model, they will need to run them on the standardized method. Having a common platform, which allows you to compute both at the same time and efficiently orchestrate the distributions of your positions across one calculation and the other, allows you to run your infrastructure in a much more effective and cost efficient way, while reducing operational risk.
There are also technical overlaps in the way these computations are run. If you look at SA-CCR and the FRTB standardized approach, the methods are different, but the principles of aggregation are very similar. You also have the same types of requirements in terms of attributing the capital from all these sources—credit risk, market risk, CVA charge—down to individual business lines, or to an individual trade.
“In the end, it is about building an enterprise-wide dashboard, which offers a complete picture of the essential parameters for a bank’s profitability—capital, liquidity, and valuation adjustments.”
Q. Are there additional uses or benefits this engine can support such as customization or support of internal models?
A. The main idea is building a robust framework. Because it is well thought out, because you have proper management of data and computations, you have an engine that can adjust to new requirements, new regulations, or new ways of managing collateral. You will have a stable and reliable infrastructure that offers consistent analysis of firm-wide positions on a daily basis, and can provide the transparency, reporting and analysis capabilities that regulators and internal stakeholders need.
A regulation engine supporting market, credit and CVA charges computations can of course support other business needs. For example, an institution can choose to extend an SA-CCR or IMM approach to deliver pre-deal counterparty limits checking to its front office desks. Similarly it can leverage the same engine for the FRTB-RSA calculation and initial margin computations under the ISDA SIMM method. It can also report standardized sensitivities across all desks. Similarly, IFRS 9 requires an enterprise-wide view of stress-testing as well, and the same infrastructure will also help achieving the Prudent Valuation requirements.
In the end, it is about building an enterprise-wide dashboard, which offers a complete picture of the essential parameters for a bank’s profitability—capital, liquidity, and valuation adjustments.
The aim is to give collateral trading units, risk managers, and traders a centralized view where they can see the current and prospective CVA, FVA, and lifetime funding costs of initial margins and capital. You will want to have a coherent view at the enterprise level of these positions, their sensitivities and have the ability to value these different costs on a pre-trade basis within front office applications.
This is why putting in place a central engine is not only an effective way of achieving regulatory compliance, but can also help the institution move a step further and develop a very strong competitive advantage.
Q. How many people are actually implementing these regulatory calculation engines? Are most people doing this now, or are they in the process of thinking about it, in your experience?
A. Some top-tier institutions have already started to build this type of capacity in-house. They may not call it a “regulatory calculation engine” per se, but these are the pioneers in that space.
A number of other institutions—in particular larger tier-two regional banks—are starting to explore this kind of vision. These are usually more in the planning stage. Medium-sized banks may actually face fewer implementation challenges than larger banks, whose vision can be hampered by a multiplicity of systems across business lines, geographies and jurisdictions, and often fragmented data ownership, and layers of spaghetti interfaces.
* For more on how financial institutions are breaking down silos to integrate data into these regulatory calculation engines, see the first post in our two-part series here. You can also watch the on demand webinar on SA-CCR – Understanding Implementation Challenges for an Effective Adoption Plan here